Our projects
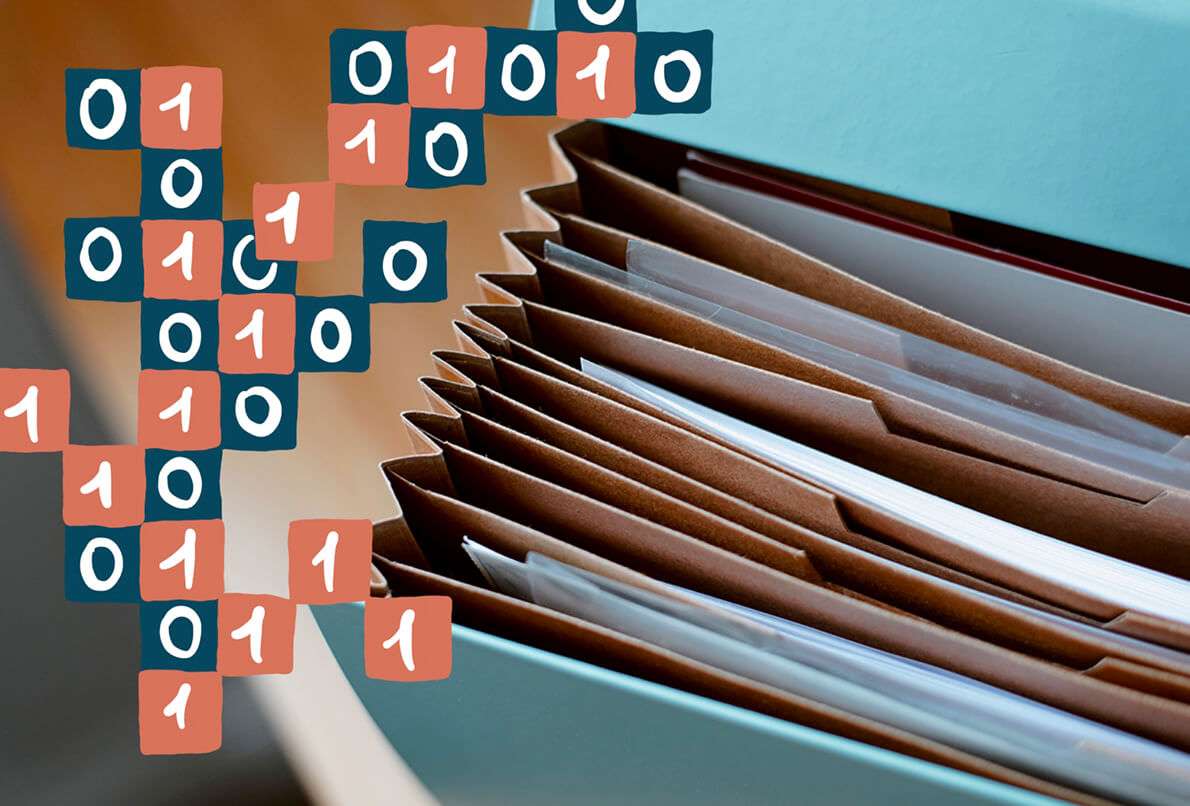
Like most back-office processes, in a banking context, mortgage verification activity is a slow and repetitive task. It requires the reading of notarized deeds, technical reports and other meaty documents for data extraction, activities that take hours.
Thanks to Robotization techniques, it is possible to automate the process entirely, avoiding errors and recovering the backlog accumulated over months.
Results
+ 15% FTE* recovered over 9 months (*full time equivalent)
+ 100% Mortgage deeds checked automatically every day
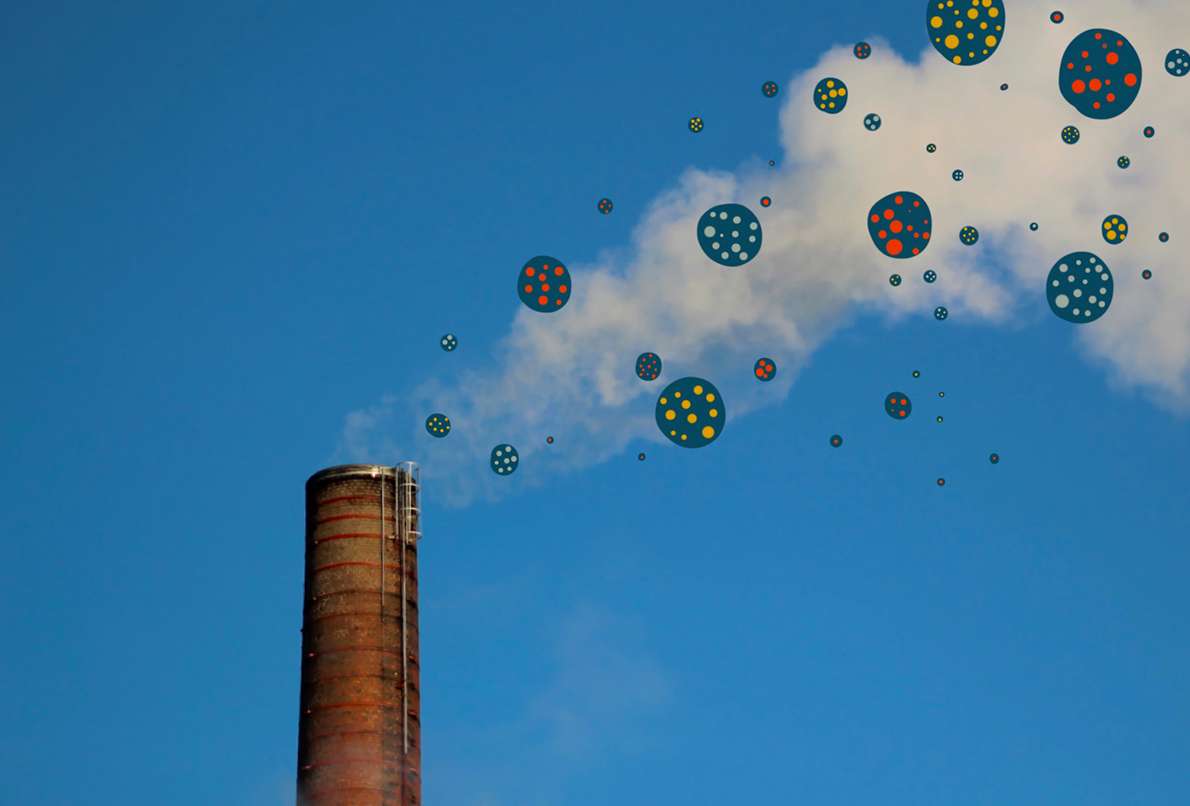
The development of CO2 capture solutions involves complex experimentation that is often left to the manual activity and experience of an operator. To make this step more effective, hybrid AI models can be applied to speed up the process.
To improve the calibration of the physical model, an integration is done with a custom model in python (hybrid model) that allows generating representative synthetic datasets that can be used to train surrogate models.
Results
– 40% Simulation time
– 12% Calibration errors
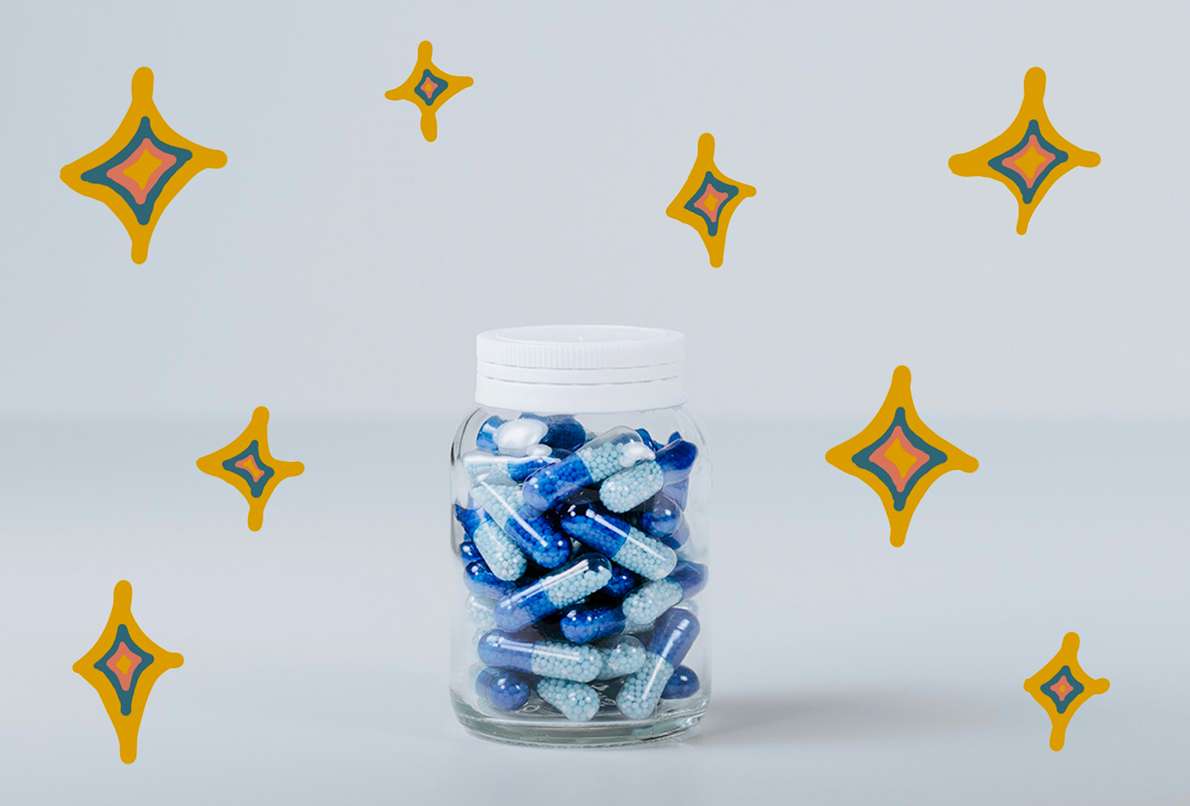
A pharmaceutical company needs to keep its production equipment always clean, in compliance with health regulations. However, the planning of cleaning shifts can run into multiple limitations. Making use of AI makes it possible to reduce the time spent on this activity and any associated error, while also simplifying the communication of planned shifts.
The AI algorithm schedules cleaning shifts based on production planning, within the constraints of cleaning needs, available resources, and related skills.
Results
– 25% FTE* on the cleaning shift planning process (*full time equivalent)
– 100% Planning errors due to overlapping shifts (or failure to meet constraints)
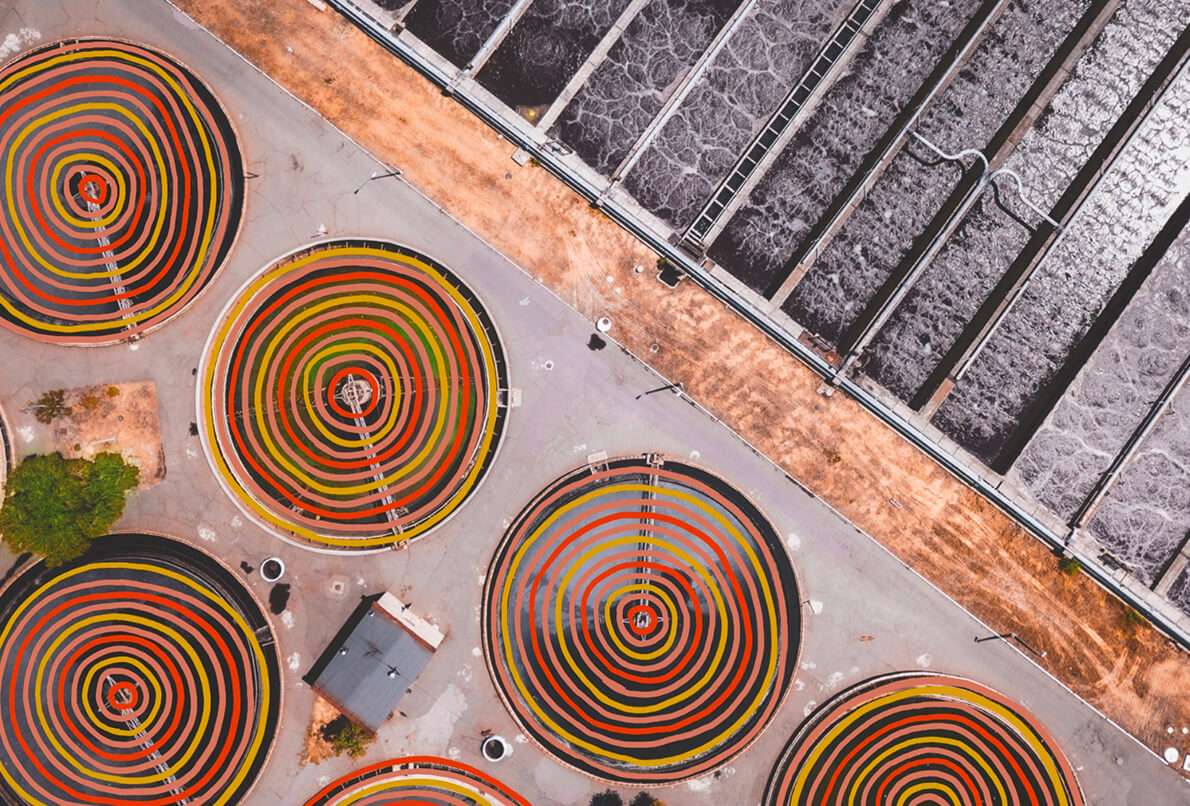
Most wastewater treatment plants in Italy lack systems for aeration management, often characterized by feedback or fixed set-point controls. Thus, spikes in pollutants can hardly be prevented, resulting in fines and penalties.
Through Model Predictive Control (MPC), it is possible to control the system by predicting its evolution and adjust oxygen in advance, depending on the expected load.
Results
– 33,7% Ammonia output compared to the previous controller
– 8% Total nitrogen compared with the previous controller
– 16% Energy savings achieved